Software/Datasets
OpenGV
Link: https://github.com/laurentkneip/opengv
Documentation: https://laurentkneip.github.io/opengv
License: FreeBSD
Precompiled Windows Matlab mex-library:
Download here!
Precompiled Mac OSX Matlab mex-library:
Download here!
Please cite the following paper if using the library:
L. Kneip, P. Furgale, "OpenGV: A unified and generalized approach to real-time calibrated geometric vision", Proc. of The IEEE International Conference on Robotics and Automation (ICRA), Hong Kong, China. May 2014. (PDF)
For more references, in particular about the algorithms that are included in OpenGV, please visit our research page on geometric camera pose estimation algorithms.
polyjam
Link: https://github.com/laurentkneip/polyjam
Documentation: click here
License: GPL
polyjam is a C++ library for setting up algebraic geometry problems and generating efficient C++ code that solves the underlying polynomial systems of equations. It notably does so by applying the theory of Groebner bases. polyjam is the driving force behind OpenGV, and all of MPL's geometric computer vision algorithms that involve the solution of multivariate polynomial equation systems contain solvers generated by this library. Problems of such form may be required in many engineering disciplines, which is why the tools provided through this library are of potentially broad applicability. Please read the documentation for in-depth user instructions.
P3P
Link: click here
Documentation: Instructions are now contained in the package
License: FreeBSD
This is the original Matlab/C++ code for the P3P algorithm of Prof. Kneip. It is the state-of-the-art solution to the absolute pose problem, which consists of computing the position and orientation of a camera given 3 image-to-world-point correspondences. Execution time in C++ lies in the order of a microsecond on common machines. The algorithm requires normalized image points, and therefore requires the camera to be intrinsically calibrated. Note that the algorithm is also contained in OpenGV.
It you use this algorithm, please cite the paper:
L Kneip, D Scaramuzza, and R Siegwart. A novel parametrization of the perspective-three-point problem for a direct computation of absolute camera position and orientation. In Proceedings of the IEEE Conference on Computer Vision and Pattern Recognition (CVPR), Colorado Springs, USA, June 2011. (PDF)
faP4P
Link: https://github.com/laurentkneip/faP4P
Documentation: Instructions should be contained in the Readme of the package
License: GPL-2.0
Open-source release of uncalibrated P4P algorithm with unknown aspect ratio, implemented with polyjam. The P4P algorithm with unknown aspect ratio basically enables to find the pose of a camera in parallel to unknown (and distinct!) focal lengths. While most consumer cameras have square pixels, cameras in scientific or industrial domains can come with rectangular pixels. Note that the algorithm is an implementation of the paper "A Novel Solution to the P4P Problem for an Uncalibrated Camera" by Yang Guo, published in 2013 in J Math Imaging Vis (2013) 45:186–198. It is indeed a jewel of geometric vision, and makes use of the dual of the image of the absolute conic to quickly reduce the problem to finding the intersection of three conics, the same problem that needs to be solved for the generalized P3P algorithm.
RBRIEF
Link: https://github.com/laurentkneip/rbrief
Documentation: See OpenCV-BRIEF interface (click here)
License: FreeBSD
A modification of the BRIEF descriptor permitting an online rotation of the extraction pattern (useful if some knowledge of the orientation around the camera principle axis is given). The interface is OpenCV compatible, and extended by two further functions:
setRotationCase( double rotation ): sets a rotated pattern from the base pattern
freezeRotationCase(): transfers the rotated pattern to the base pattern
SSS Benchmarks
Link: https://mpl.sist.shanghaitech.edu.cn/SSSBenchmark/SSSMPL.html
Documentation: See GitHub for tools usage (click here)
License: Creative Commons License & MIT
A set of synthesized realistic visual semantic SLAM dataset and benchmark with open source codes for customizing dataset. The indoor-scenario-oriented dataset includes RGBD, stereo, depth, semantic map, IMU, obejct model and location, groundtruth and hierarchical structure for both visual SLAM and neural network researches.
If you use this dataset, please cite the work:
Yuchen Cao, Lan Hu, and Laurent Kneip. Representations and benchmarking of modern visual slam systems. MDPI Sensors, 20:2572, 2020. (PDF)
Objet pose estimation
Paper: RGB-based Category-level Object Pose Estimation via Decoupled Metric Scale Recovery, J Wei, X Song, W Liu, L Kneip, H Li, and P Ji, IROS'24 (preprint, video)
Link: https://github.com/goldoak/DMSR
License: MIT
Brief: Most recent camera-based category-level object pose estimation methods depend on RGB-D depth sensors. RGB-only methods provide an alternative to this problem yet suffer from inherent scale ambiguity stemming from monocular observations. This work proposes a novel pipeline that decouples the 6D pose and size estimation to mitigate the influence of imperfect scales on rigid transformations. Specifically, it leverages a pre-trained monocular estimator to extract local geometric information, mainly facilitating the search for inlier 2D-3D correspondence. Meanwhile, a separate branch is designed to directly recover the metric scale of the object based on category-level statistics. Finally, RANSAC-PnP robustly solves for the 6D object pose.
Paper: AEPnP: A less-constrained EPnP solver for pose estimation with anisotropic scaling, J Wei, S Leutenegger, and L Kneip, ECCV'24 [workshop] (paper)
Link: https://github.com/goldoak/AEPnP
License: MIT
Brief: Perspective-n-Point (PnP) stands as a fundamental algorithm for pose estimation in various applications. AEPnP is a new approach to the PnP problem with relaxed constraints, eliminating the need for precise 3D coordinates, which is especially suitable for object pose estimation where corresponding object models may not be available in practice. Built upon the classical EPnP solver, it has the ability to handle unknown anisotropic scaling factors in addition to the common 6D transformation. Through a few algebraic manipulations and a well-chosen frame of reference, this new problem can be boiled down to a simple linear null-space problem followed by point registration-based identification of a similarity transformation.
Dynamic Event Camera Calibration
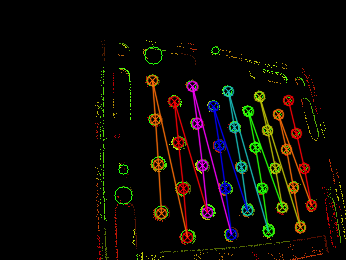
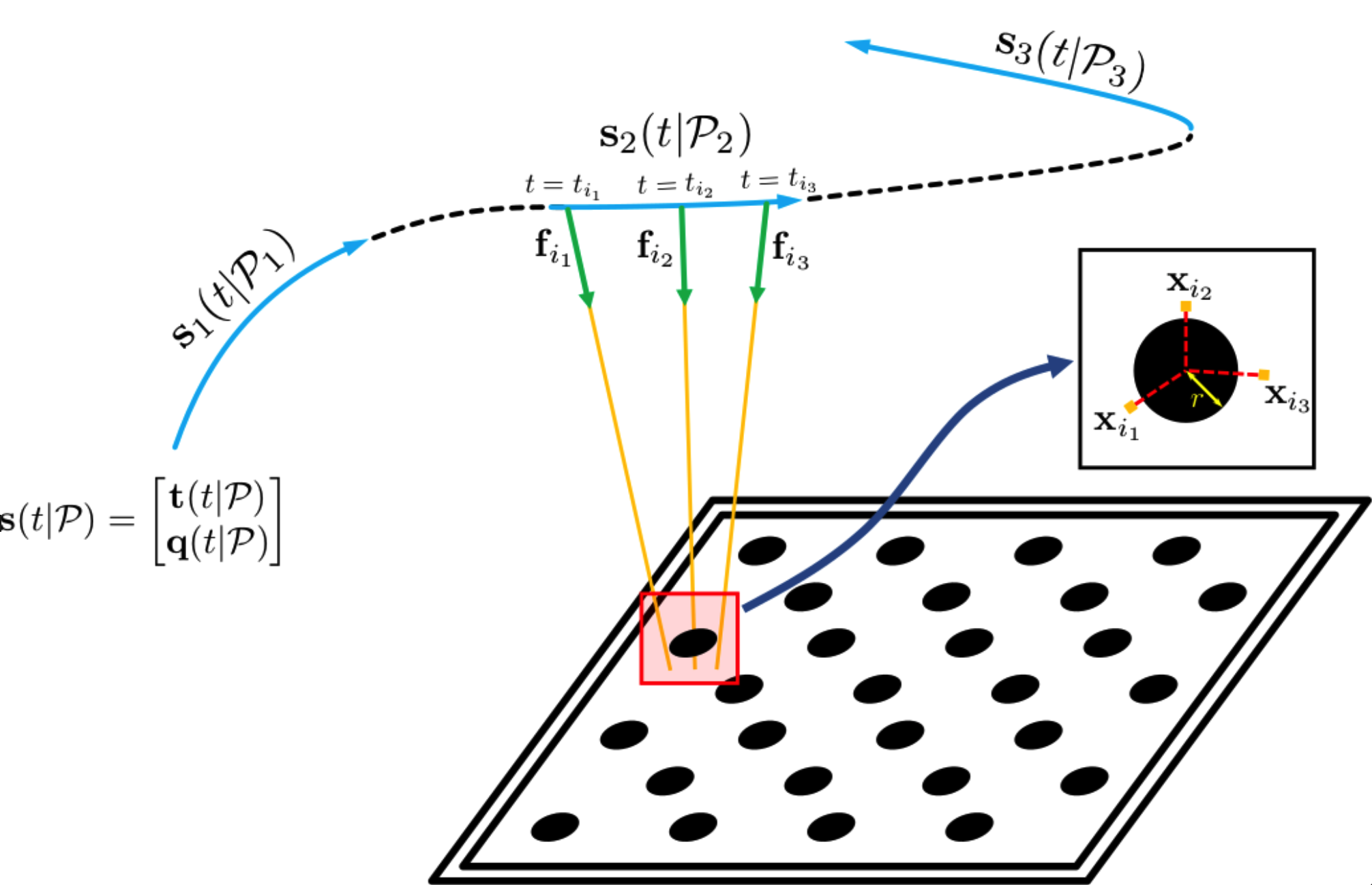
Link: https://github.com/MobilePerceptionLab/EventCalib
Documentation: Instructions are contained in the package
License: Apache
Camera calibration is an important prerequisite towards the solution of 3D computer vision problems. Traditional methods rely on static images of a calibration pattern. This raises interesting challenges towards the practical usage of event cameras, which notably require image change to produce sufficient measurements. The current standard for event camera calibration therefore consists of using flashing patterns. They have the advantage of simultaneously triggering events in all reprojected pattern feature locations, but it is difficult to construct or use such patterns in the field. We present the first dynamic event camera calibration algorithm. It calibrates directly from events captured during relative motion between camera and calibration pattern. The method is propelled by a novel feature extraction mechanism for calibration patterns, and leverages existing calibration tools before optimizing all parameters through a multi-segment continuous-time formulation. As demonstrated through results on real data, the provided calibration method is highly convenient and reliably calibrates from data sequences spanning less than 10 seconds. A circular pattern calibration board is the only requirement.
When using this tool, please cite the work:
Kun Huang, Yifu Wang, and Laurent Kneip. "Dynamic Event Camera Calibration." 2021 IEEE International Conference on Intelligent Robots and Systems (IROS). IEEE, 2021. (Youtube Bilibili PDF)
VECtor benchmark: A Versatile Event-Centric Benchmark for Multi-Sensor SLAM
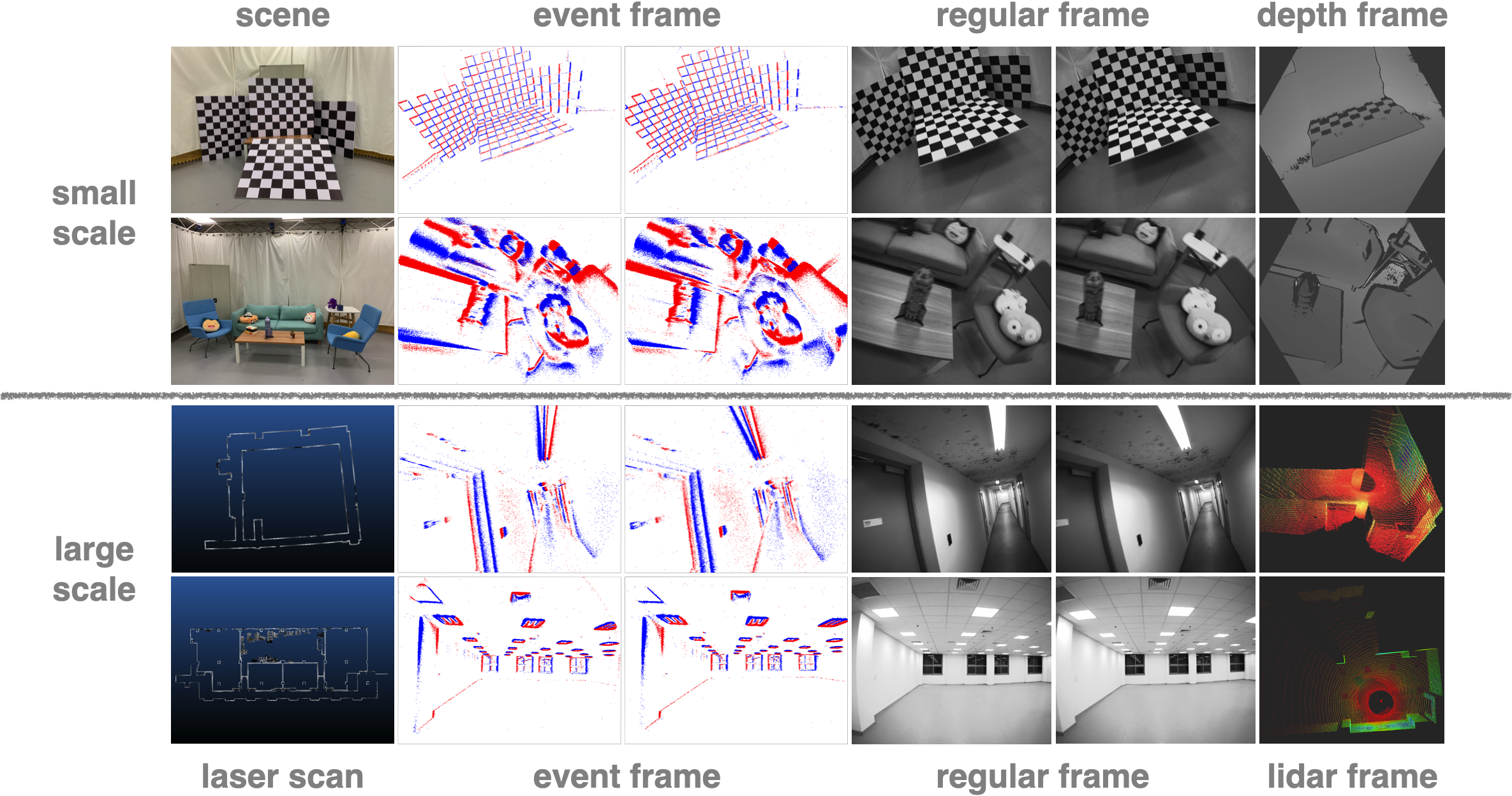
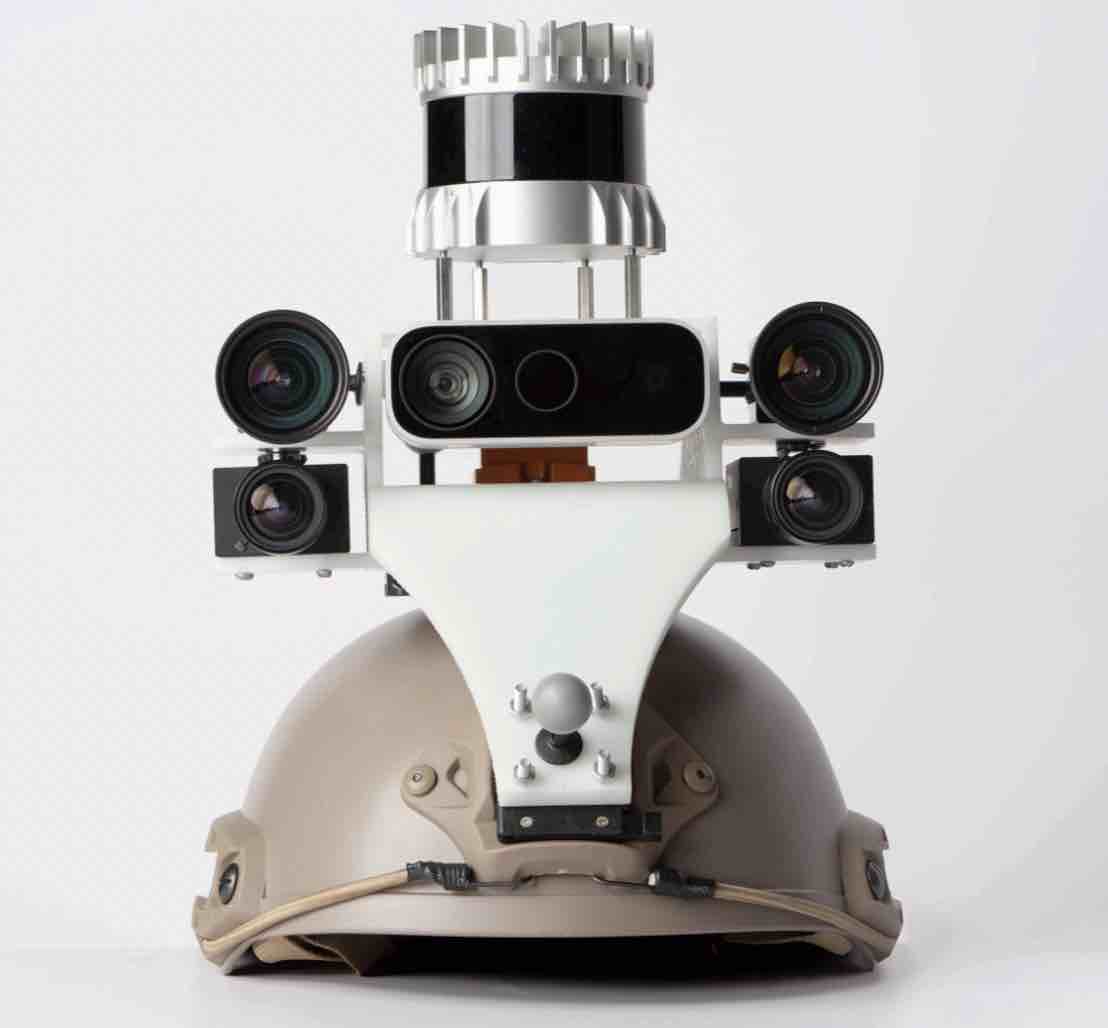
Main site: https://star-datasets.github.io/vector/
PDF: https://arxiv.org/pdf/2207.01404.pdf
Supp.: https://star-datasets.github.io/vector/assets/pdf/supplementary_material.pdf
Online presentation (youtube): https://youtu.be/WZMeKwhj434
Online presentation (bilibili): https://www.bilibili.com/video/BV1kd4y1B7Ug
Calibration Toolbox: https://github.com/mgaoling/mpl_calibration_toolbox
Dataset Toolbox: https://github.com/mgaoling/mpl_dataset_toolbox
Synchronization Toolbox: https://github.com/sjtuyuxuan/sync_toolbox
The complete set of benchmark datasets is captured with a fully hardware synchronized multi-sensor setup containing an event-based stereo camera, a regular stereo camera, multiple depth sensors, and an inertial measurement unit. All sequences come with ground truth data captured by highly accurate external reference devices such as a motion capture system. Individual sequences include both small and large-scale environments, and cover the specific challenges targeted by dynamic vision sensors (high dynamics, high dynamic range).
When using this dataset or any of its tools, please cite the work:
L. Gao, Y. Liang, J. Yang, S. Wu, C. Wang, J. Chen, and L. Kneip. VECtor: A Versatile Event-Centric Benchmark for Multi-Sensor SLAM. Robotics and Automation Letters, 7(3):8217–8224, 2022
New frameworks for extrinsic calibration of multi-perspective and multi-sensor systems
Paper: Accurate calibration of multi-perspective cameras from a generalization of the hand-eye constraint, Y Wang, W Jiang, K Huang, S Schwertfeger and L Kneip, ICRA'22 (link)
Link: https://github.com/mobileperceptionlab/multicamcalib
Brief: Calibration using a variant of hand-eye calibration. Requires external motion capture system to get chess-board poses. Documentation in Readme.
License: Apache License 2.0
Paper: Multical: Spatiotemporal Calibration for Multiple IMUs, Cameras and LiDARs, X Zhi, J Hou, Y Lu, L Kneip, and S Schwertfeger, IROS'22 (link)
Link: https://github.com/zhixy/multical
Brief: Calibration using multiple chess-boards detected and optimized by both LiDAR and cameras.
License: BSD-3
Geometric registration methods for event cameras
Paper: Event-based Visual Odometry on Non-holonomic Ground Vehicles, W Xu, S Zhang, L Cui, X Peng and L Kneip, 3DV'24 (preprint, video)
Link: https://github.com/gowanting/NHEVO
Brief: Internal solver to find the rotational velocity of an Ackermann vehicle from event streams.
License: Apache 2.0
Paper: Cross-Modal Semi-Dense 6-DoF Tracking of an Event Camera in Challenging Conditions, Y Zuo, W Xu, X Wang, Y Wang, and L Kneip, TRO'24 (paper, video (from related conf. paper))
Link: https://github.com/zyfff/Canny-EVT/
Brief: Tracking of an event camera on top of a semi-dense map generated by a regular frame-based camera.
License: Apache 2.0
Paper: A 5-Point Minimal Solver for Event Camera Relative Motion Estimation, L Gao, H Su, D Gehrig, M Cannici, D Scaramuzza, and L Kneip, ICCV'23 (link, video)
Link: https://mgaoling.github.io/eventail_iccv23/
Brief: Exact parametrization of line-generated event cluster under constant linear velocity. Minimal 5-event solver.
Paper: An n-point linear solver for line and motion estimation with event cameras, L Gao, D Gehrig, H Su, D Scaramuzza, and L Kneip, CVPR'24 (preprint, video )
Link: https://mgaoling.github.io/eventail/
Brief: Exact parametrization of line-generated event cluster under constant linear velocity. Fast, linear version of previous ICCV'23 solver (500x speed-up!! applicable to both minimal and non-minimal case!!). Note that the software link is the same, the webpage contains all eventail solvers.
Paper: EVIT: Event-Based Visual-Inertial Tracking in Semi-Dense Maps Using Windowed Nonlinear Optimization, R Yuan, T Liu, Z Dai, Y-F Zuo, and L Kneip, IROS'24 (paper, video)
Link: https://github.com/peteryuan123/CANNY_EVIT
Brief: Inertial-supported extension of Canny-EVT (see above). Also proceeds by registering semi-dense point clouds against time-surface map (TSM). Adds inertial measurements to increases performance in highly dynamic situations while keeping the rate at which TSMs are processed low.
Paper: GS-EVT: Cross-Modal Event Camera Tracking based on Gaussian Splatting, Liu et al., ICRA'25 (preprint, video)
Link: https://github.com/ChillTerry/GS-EVT
Brief: Novel map-based event camera tracking framework. Similar to Canny-EVT and EVIT (see above), it relies on prior maps generated from regular camera images. However, GSEVT operates directly on top of gaussian splatting permitting efficient, geometry-independent novel view synthesis.
Optical Camera Communication with Event Cameras
Paper: Motion-aware optical camera communication with event cameras, H. Su, L. Gao, T. Liu, and L. Kneip, ICRA'25 and RAL (preprint, video)
Link: https://github.com/suhang99/EventOCC
Brief: Novel optical camera-based system to receive data from digital screens via visible light. In order to address bandwidth and dynamics-related limitations of CMOS cameras, the introduced system utilizes an event camera for dynamic visual marker-based tracking, localization, and communication. The event camera's unique capabilities mitigate issues related to screen refresh rates and camera motion, enabling a high throughput of up to 114 Kbps in static conditions, and a 1cm localization accuracy with 1% bit error rate under various camera motions.